- Undergraduate
Bachelor's Degrees
Bachelor of ArtsBachelor of EngineeringDual-Degree ProgramUndergraduate AdmissionsUndergraduate Experience
- Graduate
Graduate Experience
- Research
- Entrepreneurship
- Community
- About
-
Search
All Thayer News
Dartmouth Engineering Study Shows Machine Learning Can Help Airlines Recover Faster After Disruptions
Dec 10, 2024 | by Catha Mayor
If not recovered quickly, disrupted aircraft schedules can come at a high cost in terms of money, time, and airline reputation. A Dartmouth Engineering research team has shown that when carefully applied, machine learning tools can save precious time—and therefore money—in the decision-making process for aircraft scheduling recovery.
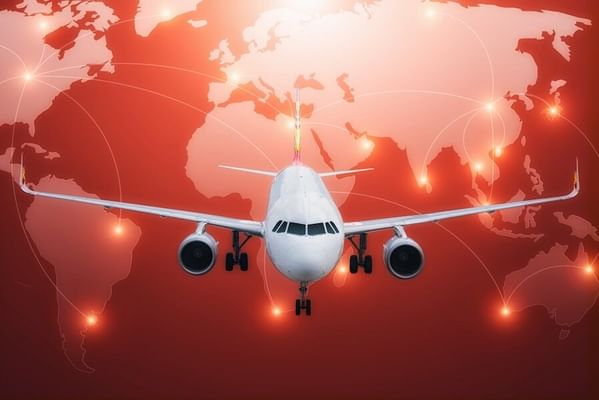
Image credit: Yok Piyapong
"We're talking about hundreds of thousands of dollars a day in potential delay and cancellation costs," said Vikrant Vaze, professor of engineering at Dartmouth and author on the study published today in the European Journal of Operational Research. "Because let's say you're waiting to board your flight, and they say, 'We don't know what's happening with your flight, or with the other five-hundred flights today, but just wait thirty minutes while I run this algorithm that might be able to tell us what to do. Meanwhile, all the passengers are stranded, all the crews are waiting, and all the aircraft are stuck."
Although Vaze's team's algorithms have long been able to find solutions to these problems, running them simply took too long, and using traditional methods to speed them up tended to degrade the results.
"Airlines have struggled forever to solve recovery problems quickly," explained Vaze. "Our study shows that machine learning enables our algorithms to produce nearly the best possible answers within one or two minutes—as opposed to taking much longer and getting only a mediocre solution."
For the field of operations research, this is new territory because machine learning has been missing the mark when it comes to solving these kinds of constrained problems. The trick, said Vaze, is to strategically limit the set of tasks that it solves by itself.
"We let machine learning go first just to narrow things down. Then our algorithm takes over to focus only on the most promising options. Basically, when we're finding the needle in the haystack, machine learning can tell us which haystacks are totally useless. But knowing exactly where it is in those few promising haystacks is not something machine learning can do very easily.
"If you told me that tomorrow things are going to go down this way, I can give you a fantastic solution without machine learning. But when things go bad, we have no time," continued Vaze. "It's not perfect, but when given a range of possible options—which are more likely to give you lower costs, faster recovery, greater revenue, all the things we care about in operations research—machine learning can help spit out a good solution very quickly."
The team's approach can also be useful in any situation where speed is a crucial factor.
"The broad area we're talking about is routing. Planes are routed through flights. So instead of this flight to this flight to this flight, you can say, this FedEx driver should go here, then here, then here. Or this Instacart driver. Routing is everywhere."
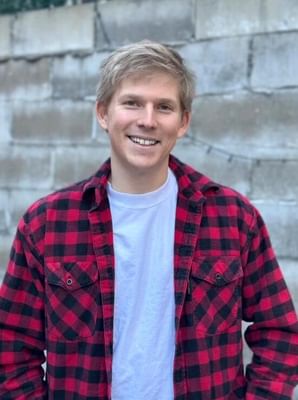
Second author Nolan Sankey '21 Th'22. (Photo courtesy of Nolan Sankey)
Also of note is that this particular study came from the combined work of a professor, an alum, a PhD student, and an undergraduate student at Dartmouth.
"This is the first time, for me, that we have an undergrad as a key author on a top journal paper," remarked Vaze. "I thought it would just be a nice educational experience for him, but then he kept going and started generating results. My PhD student, Navid Rashedi, developed the idea and is first author, but there was a period where Nolan [Sankey] totally took over and did everything. And then he handed it back to us, and we took it from there."
"I was looking to join a research team during a term when I was taking classes off and ski patrolling at the Dartmouth Skiway," says Sankey. "Developing this novel approach with Professor Vaze and Navid Rashedi was a unique opportunity. Professor Vaze brought industry-leading expertise to the project, yet I was given significant autonomy to program key portions of the optimization algorithm and was encouraged to bring my ideas to the group to improve the results."
Sankey worked with Vaze’s team from 2020–2021. He earned his AB from Dartmouth in 2021, and his BE from Thayer in 2022. He now works as a mechanical engineer at Ultima Genomics in New York.
For contacts and other media information visit our Media Resources page.