- Undergraduate
Bachelor's Degrees
Bachelor of ArtsBachelor of EngineeringDual-Degree ProgramUndergraduate AdmissionsUndergraduate Experience
- Graduate
Graduate Experience
- Research
- Entrepreneurship
- Community
- About
-
Search
All Thayer News
Artificial Intelligence by Intention
May 20, 2024 | by Michael Blanding | Dartmouth Engineer
Eugene Santos Jr., the Sydney E. Junkins 1887 Professor of Engineering, looks at how artificial intelligence (AI) can help us make better decisions in fields ranging from national security to healthcare delivery to cancer research—and he believes we hold the power to improve AI in a way that truly benefits human lives.
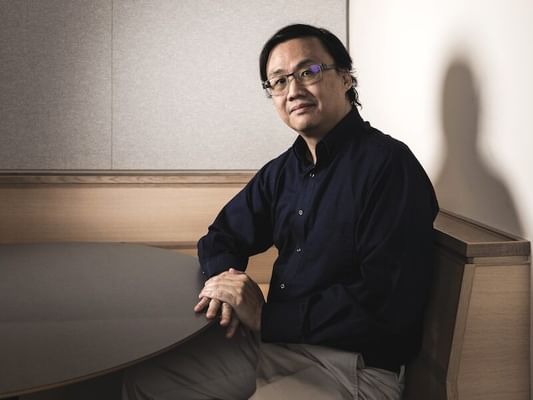
Eugene Santos Jr., Dartmouth's Sydney E. Junkins 1887 Professor of Engineering
We all know what it's like: sitting down to watch a movie, but scrolling needlessly because Netflix keeps recommending films we would never watch. Then someone chimes in that ChatGPT could fix all this.
"People are hallucinating together with ChatGPT," Professor Eugene Santos Jr. says, only half-jokingly. "Even when ChatGPT's response seems nonsensical to you, some generative AI believer will say it's you who need to broaden your thinking."
It's not that Santos is an AI pessimist. Rather, he has spent his career pursuing AI and knows problems run deeper than mere misfires in its predictive capabilities—it's about the system misunderstanding our intentions. With AI increasingly integrated into our everyday tools, from navigation apps to smart phones and home devices, Santos believes developing AI that better interprets human intention could transform an imperfect tool into a more finely calibrated virtual assistant.
"We're always trying to figure out each other's intentions," Santos says. "And part of that is understanding the sequence of decisions another person would make, how their arguments are unfolding in their head."
Humans naturally anticipate this sequence when interacting with others and build real-time mental models to help make sense of another's words and behavior—and ask follow-up questions to clarify when they don't understand. "If nothing else, we can communicate better, because you have both sides starting to gain better knowledge rather than going in with implicit assumptions," Santos says.
Current AI models often falter in that capacity, operating through frameworks that look for the closest match, the next most likely word or concept based on training on vast amounts of data. For example, when you accidentally click on The Fast and the Furious, Netflix uses that input to make its next movie recommendation, lacking the ability to realize that you actually meant to click on the adjacent Pride & Prejudice.
Generative AI models such as ChatGPT fare better, says Santos, since they allow more interplay with the system. For instance, if you asked ChatGPT about "Ford" with the hope of getting more information about "Gerald Ford" and it instead tells you about the car company, you can clarify you meant the former president. At the same time, large language models are also notorious for returning so-called "hallucinations"—incorrect information with the ring of truth based on what the model is trained to believe the user wants to hear.
One key to improving AI, Santos says, lies in creating models that are better at interpreting people's behaviors and the intent behind their queries. This would allow AI to refine its understanding of human thought processes and deliver more accurate information to satisfy those requests.
"The idea is that as the AI observes you, whatever information you are generating—even your facial expressions when we get that far—forms these networks of concepts and creates a running model over time of how you've interacted with it," he says. Eventually, an AI model may even be able to tell when your reasoning doesn't make sense and correct wrong assumptions before carrying out a task.
Other crucial factors to better AI are the engineers or computer scientists themselves. With AI shaping many facets of our lives, Santos says it is just as important that the people who develop integrated technology have a deeper, human-centered understanding of the profound societal implications of their work.
Santos, who also serves as director of Thayer's Master of Engineering Program, was central to the launch this winter of Dartmouth's first online master of engineering in computer engineering. The first fully online Ivy League degree, it focuses on intelligent systems, the foundational technology for AI. And it's offered online for a reason: Diversity matters in the realm of AI. For instance, speech patterns, facial features, gender norms, and cultural practices all shape, impact, and influence human thought and behaviors engineers and developers must understand and consider.
"Computer engineering is an area that's accelerating rapidly," he says, "and the flexibility of an online program helps us reach a broader, more diverse group of engineers—people we may not have been able to reach before—and that could mean stronger AI tools for everyone."
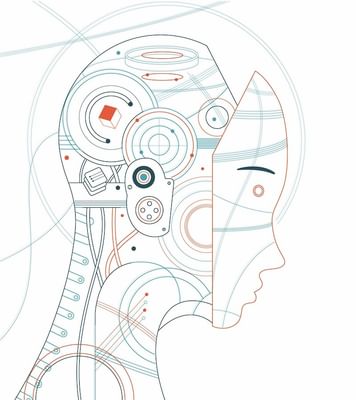
(Illustration by Harry Campbell)
Santos was exposed to computers early in life. His parents, both professors at Youngstown State University, were computer scientists by training—his mother an electrical engineer and his father a mathematician. "I got really lucky in that my dad brought home from the university the first teletype machine connected to a mainframe over the phone," he says. "They never pushed anything on me, but when you have this cool stuff that flows through your home, you can't avoid it."
Santos was instantly hooked, wondering even then how computers could be made more intelligent.
In the 1980s, he began studying expert systems, an early precursor to AI. During graduate studies at Brown, his interests diverged from most computer scientists studying AI. Instead of training AI to make better predictions, Santos was more interested in learning why AI made the predictions it did. After stints at the Air Force Institute of Technology in Ohio and the University of Connecticut, in 2005 he joined Dartmouth's engineering faculty, where he delved deeper into his research.
By creating AI models that focus on the intent behind decision-making, Santos has also developed a better understanding of how humans make decisions in complicated situations. "We don't work in isolation, we work in groups and organizations and influence each other," he says. AI can be particularly helpful in analyzing how intentions and decisions by some individuals affect those of others.
In one project with his sister, Eunice E. Santos, dean of the University of Illinois at Urbana-Champaign School of Information Sciences, he helped analyze the political collapse of Somalia, studying how the country went from a "nation state to a failed state to a pirate state," as he puts it. "We were able to explain shocks to the system and how it all evolved," says Santos, who coauthored a paper on "Modeling Social Resilience in Communities."
He applied similar models to analyze how countries respond to natural disasters or how an organization becomes fanatical. As these models become more refined, he says, they could be used by policymakers to prevent, intervene, or better respond to these kinds of societal crises. "If we understand how it can happen and what the impacts are, we can ideally change policies early enough so we don't get to that crisis point," he says.
In another project, he analyzed a fascinating phenomenon in social science known as "emergence," in which an unexpected outcome emerges from seemingly predictable sources. For example, during a trial, every member of the jury may enter deliberations with the belief that the defendant is guilty, but after deliberating with others, the jury may end up with a unanimous "not guilty" verdict. "Each person who is making their decision may have a certainty level where 'guilty' is slightly higher than 'not guilty,'" Santos says. However, the factors that led to that belief may differ for each juror—so as the jury compares notes, individuals disprove each other's theories, flipping each juror to a not guilty verdict.
These types of outcomes are notoriously difficult to detect. In one real-world scenario, Santos analyzed results of the 2015 UK election, in which the polls predicted an even split for the Conservative and Labor parties, but the Conservatives won by a seven-point margin. Many pundits speculated the unexpected result was based on bad polling or respondents lying about their true voting intentions. But Santos' team built an AI model showing the outcome was a case of emergence—with certain demographic groups who felt more strongly about their decision prevailing over those who were more ambivalent on election day.
"The polls were too simplistic—they weren't considering the interactions between different groups that drove the results in a different direction," Santos says. Not only did his team's model more accurately predict the outcome than the polls, it also showed it could be applied to other situations where social influence could affect collective intelligence.
"AI may talk to the decision maker, but the human still has to push the button."
Professor Eugene Santos Jr.
Santos himself is naturally inclined to take a creative approach to problems, says PhD student Clement Nyanhongo '17 Th'18. "Sometimes you find yourself going in one direction, and Professor Santos is very good at giving you other ways to think about the problem and consider it from diverse perspectives," says Nyanhongo. "He encourages you to take the space and time to explore it to get a more holistic view."
Nyanhongo's research looks at how teams function, examining the interactions between members to get a better handle on how individual strengths and weaknesses affect the team's success overall. "When we evaluate teams, it's difficult to assess the contributions of individuals without introducing subjectivity and bias," he says. An AI approach can apply a more mathematical perspective to the analysis, with the aim of providing crucial information to help teams improve.
Santos' decision-based approach to AI isn't applicable only to politics and social interactions. He is also turning it to scientific discovery—using artificial intelligence to accelerate efforts to cure cancer. The challenge, he says, isn't a lack of scientific research—it's the lack of capacity to rapidly sift through the mountainous clinical data and research findings from thousands of labs and hospitals across the world to make novel connections about what works, could work, or doesn't work in cancer treatment.
"How can we bring all of that knowledge together and discover new directions to explore?" he says.
Clinicians rely on past data to develop hypotheses about the best treatments, but Santos is spearheading a more systematic approach through a $3.4 million National Institutes of Health-funded project he is leading with Dartmouth colleagues and partners from Tufts Clinical and Translational Science Institute. Santos' emphasis on decision making is ripe for the task. "It's looking at a chain of arguments that says, 'Hey, you should look at this drug because it affects this particular gene expression, and that gene expression will affect these particular changes in these organs or cells,'" he says.
By analyzing the genetic profiles of patients and the drugs they've been exposed to, he hopes to decrease the uncertainty that currently plagues cancer research and present a more educated hypothesis to researchers through the nationally funded Biomedical Data Translator Consortium. The goal is to add enough information and analysis to go live within the next year so biomedical researchers can start using it.
As an engineer and computer scientist, Santos believes we have less to fear from AI than portrayed in media and popular culture. It's up to humans to design AI systems to help us make better decisions, he says, rather than allowing systems to make those decisions for us. "People may say AI is going to start nuclear war—but that's only true if you abdicate control and give it connection to the button that fires the missiles," Santos says. "AI may talk to the decision-maker, but the human still has to push the button."
In fact, he says, AI has more potential to be a force for good. It can help augment human intelligence by giving us much more capacity to observe vast numbers of interactions and discern patterns we are not able to identify by ourselves. That, in turn, can only help us do what we do better—whether that's responding to disasters, averting political crises, or coming one step closer to curing cancer.
MICHAEL BLANDING is a Boston-based journalist whose work has appeared in WIRED, Smithsonian, The New York Times, The Nation, and The Boston Globe Magazine.
For contacts and other media information visit our Media Resources page.