- Undergraduate
Bachelor's Degrees
Bachelor of ArtsBachelor of EngineeringDual-Degree ProgramUndergraduate AdmissionsUndergraduate Experience
- Graduate
Graduate Experience
- Research
- Entrepreneurship
- Community
- About
-
Search
All Thayer News
Dartmouth Engineers Develop New Machine Learning Approach to Extract Both General and Specific Knowledge from Big Data
Feb 16, 2023 | by Catha Mayor
Dartmouth Engineering PhD candidate Chase Yakaboski and Professor Eugene Santos Jr. have devised a way of extracting knowledge from data that not only results in more certain generalizations but also accesses more reliable knowledge at the individual level. This two-tiered machine learning algorithm has a broad range of applications including for guided biomedical research and individualized medicine.
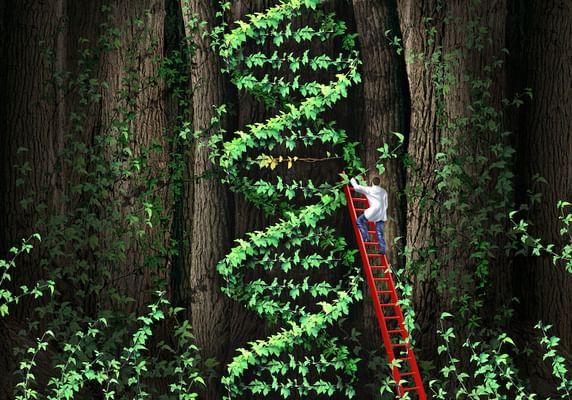
"Don't lose the trees for the forest," says first-author Yakaboski about analyzing data in a way that's valuable for the individual as well as the whole. (Image by wildpixel)
"We've threaded the needle between capturing information about the whole and capturing information about the parts," says Yakaboski, "Most people tackle it either one way or the other."
"Our goal is also about making knowledge more certain," adds Santos. "Important knowledge is derived both from the individuals and from the whole."
Yakaboski and Santos are co-authors of "Learning the Finer Things: Bayesian Structure Learning at the Instantiation Level" presented last week at the 37th Association for the Advancement of Artificial Intelligence (AAAI) Conference in Washington, DC. The authors demonstrated the utility of their approach by "learning gene regulatory networks on breast cancer gene mutational data available from The Cancer Genome Atlas (TCGA)."
"We have more patient data for breast cancer than we do for other, rarer cancer types. It's also specific, so it eliminates many potentially confounding issues," says Yakaboski. "People want to see how your algorithm performs on a sample problem, and then on some standard benchmarking datasets that are used throughout the community. And then, if you have a particular study you think is important, apply it to that as well. This three-pronged evolution shows how well your algorithm works in different computational settings."
As the ability to collect and store data continues to grow, the demand for help from artificial intelligence (AI) to manage and learn from that data grows with it.
"If you have a biomedical researcher that has a lot of data, but they don't quite know how to process that data and don't know how to elicit knowledge from that data," explains Yakaboski, "then applying what we've done can help them discover the findings they need to make a new drug or a new treatment that then directly helps the patient."
"The calculator, for instance, made math more accessible and created a more equal playing field. I think any improvement in AI is trying to do the same thing. If we can put this in the hands of a lot of diverse people and they can figure out, oh, this is actually where the insights are. Now we've saved them a ton of time to do what they're good at."
Chase Yakaboski
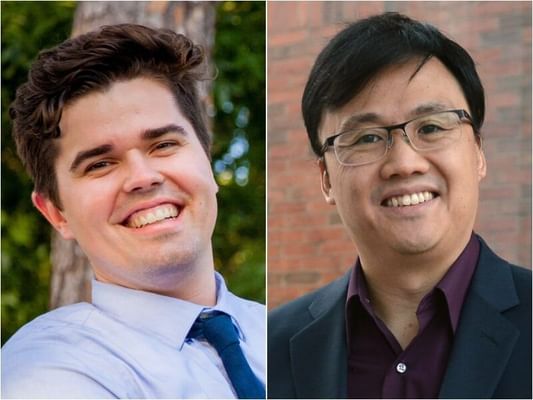
Co-authors PhD candidate Chase Yakaboski (l) and Professor Eugene Santos Jr.
"What I've been working on for the past 25 or 30 years," adds Santos, "is coming up with this model that can capture a level of fidelity that, first of all, doesn't clobber you from needing too much computation or too much time to deal with it. Because that's been one of the problems of big data. And then secondly, showing the things that we need to capture—the causality and the context and the individuality. Those didn't exist before."
Continues Yakaboski, "What we've done is collected a bunch of individual pieces of patient knowledge and fused that information together and we learned that from data. If a new individual comes in, our model is naturally dynamic. We can take their information, fuse that into the cohort, and actually reason about their individual context, which is not what a lot of other methods do."
"It's not that we're resolving the inconsistencies and contradictions, it's the inconsistencies and contradictions that make our models stronger," says Santos. "We allow for the individual to still shine through. Statistics is about the 95%. But what about the 5% left? They're important, too. Maybe try and take that into account. That's what we do."
Adds Yakaboski, "Don't lose the trees for the forest."
For contacts and other media information visit our Media Resources page.