- Undergraduate
Bachelor's Degrees
Bachelor of ArtsBachelor of EngineeringDual-Degree ProgramUndergraduate AdmissionsUndergraduate Experience
- Graduate
Graduate Experience
- Research
- Entrepreneurship
- Community
- About
-
Search
All Thayer News
Dartmouth Engineering Wins DARPA Cybersecurity Research Grant
Oct 06, 2023 | by Catha Mayor
Dartmouth Engineering professor Peter Chin will lead a four-year $4 million grant from the Defense Advanced Research Projects Agency (DARPA) as part of its Cyber Agents for Security Testing and Learning Environments (CASTLE) program. The project called, "Adaptive Hierarchical Game-theoretic RL Training for Cyber Network Defense," is a joint effort between Professor Chin's Learning, Intelligence + Signal Processing (LISP) Lab and the Network and Distributed Systems Security Lab at Northeastern University.
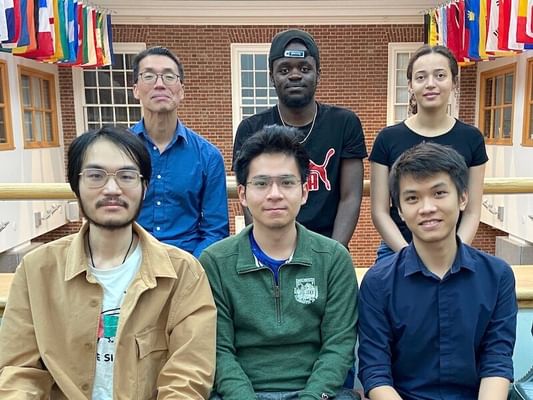
Members of the Learning, Intelligence + Signal Processing (LISP) Lab: (back row, l to r) Professor Peter Chin, Edwin Onyango '25, MS candidate Felicia Schenkelberg; (front row l to r) PhD candidates Junyan Cheng, Quang Truong, and Thang Nguyen. Not pictured: PhD candidate Allison Mann, MS candidate Zachary Gottesman, Zachary Nelson-Marois '24, Chelsea Joe '25, Iroda Abdulazizova '26, Destin Niyomufasha '26. (Photo by Catha Mayor)
"We provide expertise in RL [reinforcement learning] and game theory, and they provide expertise in cybersecurity, but we both know a good deal about each other's expertise, so it's truly a team effort," says Chin.
Chin's LISP Lab plans to develop an adaptive, hierarchical, game-theoretic RL algorithm and training framework that aims to predict and defend against future cyber attacks.
"It is of utmost importance to build a computer network that is secure, trustworthy, and responds to, and even predicts, ever-increasing cyber attacks," says Chin. "This is a significant challenge as the existing networks have grown organically over the years, often in an ad-hoc manner, and have become a complex and heterogeneous mixture of sub-networks."
This complexity makes it difficult not only to protect all possible paths of a cyber attack, but also to identify, mitigate, and pre-empt real-time attacks while maintaining normal network operations. Recent advances in deep learning—particularly in RL, a machine learning training method based on rewarding desired behaviors and penalizing undesired ones—offer hope for solutions. So far, however, models attempting to accurately anticipate adversarial attacks have fallen short of reality.
"Cyber-defense teams often fail to understand that the mitigation actions they're taking to defend the network are precisely the steps the adversaries are expecting," explains Chin. "Furthermore, while many current RL approaches still assume a static nature of the classic RL framework, our approach takes into account that an adaptive and decentralized RL framework will be necessary to combat the high sophistication level of the cyber threats of today."
Undergraduate students interested in joining this research effort are welcome to reach out to Professor Chin.
For contacts and other media information visit our Media Resources page.