- Undergraduate
Bachelor's Degrees
Bachelor of ArtsBachelor of EngineeringDual-Degree ProgramUndergraduate AdmissionsUndergraduate Experience
- Graduate
Graduate Experience
- Research
- Entrepreneurship
- Community
- About
-
Search
All Thayer News
In AI We Trust? Dartmouth Engineering Uses Unique Method to Review the Research
Feb 29, 2024 | by Catha Mayor
Engineering PhD candidate Bruno Miranda Henrique and Eugene Santos Jr., Dartmouth's Sydney E. Junkins 1887 Professor of Engineering, conducted a systematic literature review of the most important studies on trust in AI. Their unique citation analysis method not only provides a roadmap of the most significant works to date, but also critically identifies important knowledge gaps in the area of human trust in AI and, in particular, the other way around—AI trust in humans.
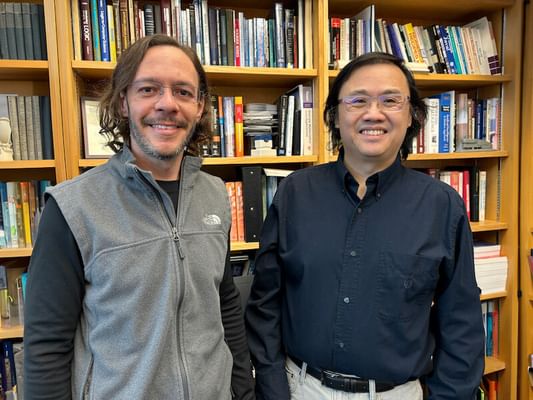
Co-authors PhD candidate Bruno Miranda Henrique (left) and Eugene Santos Jr., Sydney E. Junkins 1887 Professor of Engineering. (Photo by Catha Mayor)
Their work, presented in "Trust in artificial intelligence: Literature review and main path analysis" published last month on ScienceDirect, uses a quantitative method to organize and assess the existing body of research about achieving optimal trust levels between humans and AI systems.
"I first came in contact with the main path analysis [MPA] technique several years ago when I found a paper by P. Dereian from 1989," says Henrique, a Fulbright Scholar from Brazil. Starting with a chronological ordering of papers in a given field based on citations, the technique involves designating a main path through the network of papers that highlights the most influential works and shows how the field has evolved.
"The only problem was knowing how to build the underlying citations network," explains Henrique. "Dereian's paper wasn't clear about that. So I wrote what became a tutorial on how to build citation networks for MPA—published in Scientometrics—and applied those same techniques to this literature review."
We asked Professor Santos what the review showed about current approaches, gaps, and opportunities in the field of trust in AI:
What's the general purpose of a literature review?
If you talk to any researcher, the first thing you do is a literature review to know what the foundations are. It's meant to let everybody see—this is where everything lies, this is what's there, and help them identify the gaps. Because sometimes, what you expected to be there is not there. That can be the biggest value.
Why did you conduct this review?
I'm studying AI, not just for the sake of understanding intelligence, but to build systems that are meant to be used. We need to understand what the ramifications are, especially because it's not just about one human interacting with one AI, it's also human-AI teams. We're collaborating. We can have multiple AIs and multiple people working together towards a common goal, and to achieve that, we need to understand what trust means, which is hard to define even between human beings.
What's your working definition of AI?
My definition of AI is anything that chooses one decision from a set of possible decisions, such as making a recommendation or even taking an autonomous action. I think this power naturally illustrates both the opportunities and risks of AI.
Why is trust in AI important to the average person?
Many people don't realize that AI is already everywhere. Your cell phone is full of AI. Siri, Alexa, that's all AI. These systems are going out to the general public to use. And if you want to make, say, an effective decision support system, trust is a huge factor. We can't blindly trust it, but we can't be overly skeptical either.
What's your biggest takeaway from the review?
The fact that nobody was talking about dynamic trust calibration in AI. Even though it's a two-way street, work has pretty much solely focused on human trust in AI, and not the other way around. But modern AI systems also adapt to humans. Adjusting trust levels based on both human and AI expectations is essential. Lack of trust by humans may lead to underutilization of AI, while blind acceptance poses risks. The reverse for AI systems can lead to either ignoring the human entirely, or risking following the human over the proverbial cliff.
Our main path analysis revealed a lack of attention to the importance of two-way dynamic trust calibration within AI systems. Greater effort is needed toward developing a joint trust model based on measurable features, which considers how humans adjust to AI responses and vice-versa. Work toward achieving optimal trust levels both ways could significantly improve and enhance human collaboration with AI systems.
How do you make trust in AI a two-way street?
That, to me, is the most fun challenge. If trust is inherently a two-way street, what does the AI need to be able to do? It needs that critical thinking aspect too, to be able to question the human and understand intentions. Intentions encompass trust, and there are many layers. It involves not only what you know, but also knowing what you don't know, and being able to assess that. And then AI has to do the same thing so that both the human and the AI have just the right amount of skepticism, and know when to stop each other and ask questions.
That's where dynamic trust calibration comes in, which is something I think we take for granted. If I'm working with somebody, for example, and something happens in their life that impacts what they're thinking and what they're doing, then I'm going to recalibrate. Things are changing all the time and that dynamism has to work on both sides, for both the AI and the human.
What's next for you after this review?
This has informed us enough that we're now building dynamic trust calibration into our lab's AI decision support systems. The questions are, what can I measure from the AI and how much information do I need to pass from the AI to the human, and vice-versa.
It's a continuous feedback loop to monitor human-AI teams to say either you're diverging or you're converging too much, because the last thing you want is for one or the other or both to just say yes all the time. We need to ask, "Why are you always agreeing? Does that make sense? Or are you over-agreeing because there's something else going on?" Because they're not just collaborating on the problem, they're collaborating on trust. And they both need to use critical thinking and stay in that place of healthy skepticism, which is also important to talk about—that it's good, that it's needed, and that it should be in our minds as these tools evolve.
For contacts and other media information visit our Media Resources page.